Part 1 – Data: Your Next Big Opportunity
- Tony Camme
- July 21, 2023
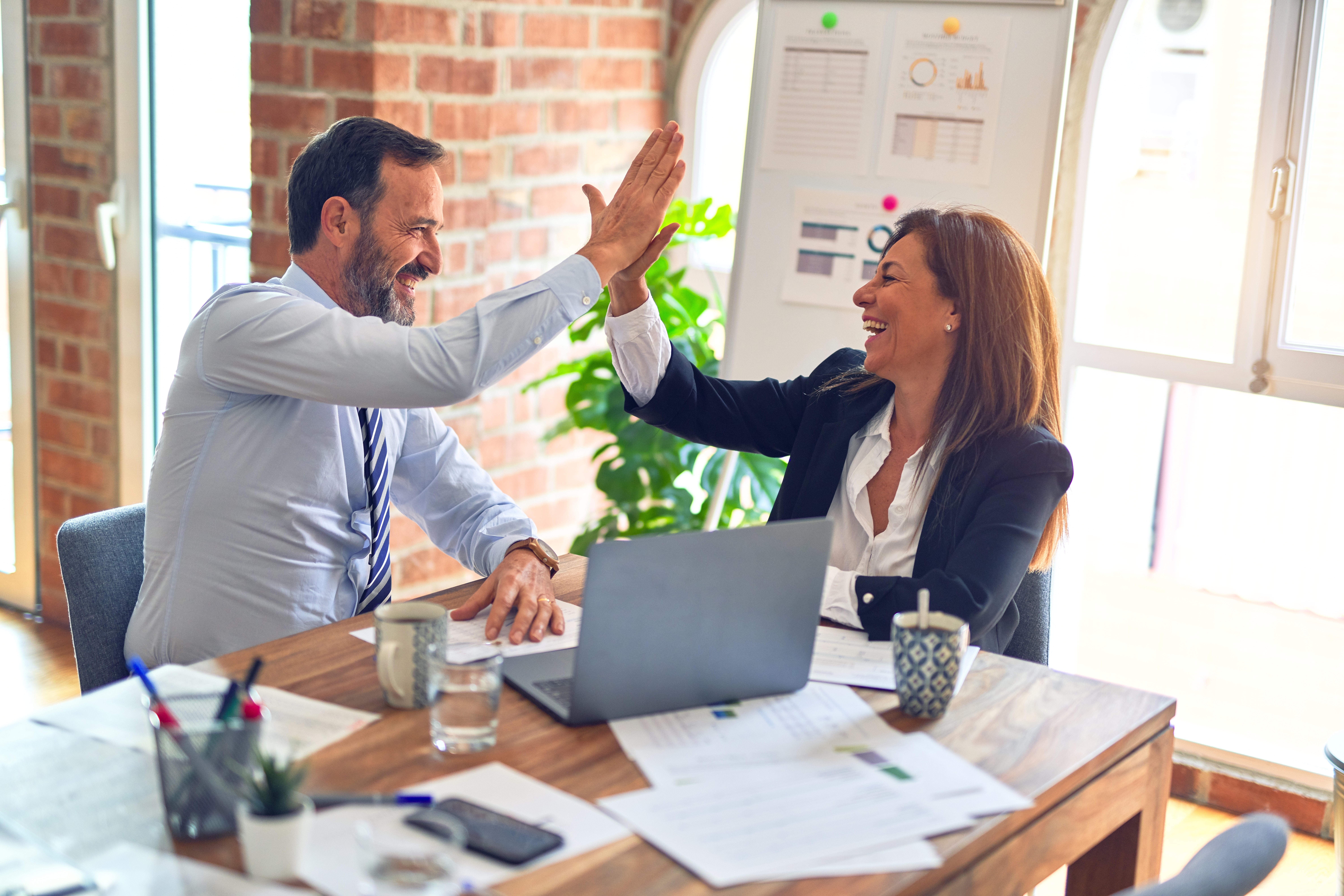
ALX Global sat down with the ExploreAI team to discuss all things data. The purpose of this Q&A was to discuss two of the newest ALX Global programs developed in partnership with ExploreAI Academy: Data Science and Data Analytics.
ExploreAI is a global data company that builds AI-driven software to help train workforces, hire talent, and sponsor learners. Its learning institution, ExploreAI Academy, provides access to data science education for people across the world to help them upskill and build long-lasting careers in data. In this new era of big data, their programs are essential.
The ensuing Q&A highlighted how massive the data industry has become. Big data is big business – companies small and large use it to influence modern ways of doing business.
A major focus of this chat was to highlight the differences between data analytics and data science, answering questions like:
- What is it like working in the data industry?
- What does it take to be successful in the data industry?
- What skills are needed to become successful in this industry?
- What course(s) are the right path for someone looking to kickstart their data career?
Here is part 01 with some of the most important bits of the Q&A:
Q: What is the difference between a data scientist and a data analyst?
A: Both roles have so many career options to choose from. Typically, we see a role in data analytics having a lot of context within a business.
As a data scientist, you can find yourself specializing as a machine learning engineer, for example, or a data engineer, but most roles are also centered around business. These are closely-related fields that are exploding at the moment.
Compared to data analysts, data scientists go a little bit further. A data scientist has the skills of a data analyst that are augmented by not only doing descriptive activities – not only saying, “How do we make sense of the data that’s in front of us?” – but also, “How can we be more prescriptive with it?”
What this means is that you not only lay the groundwork for what the data is saying, but also look ahead and decide proactively what the data could be projecting for the future. This can guide future actions businesses need to take or help them better respond to the data they currently have on hand.
That prescriptive action speaks to some more advanced statistical and computational skill sets that are often required. These are known on a day-to-day basis as things like Artificial Intelligence (AI) or machine learning. We can apply these additional skills to the data in order to model it in a way that allow us to be not only descriptive, but prescriptive as well.
So a data scientist, just like a data analyst, would be able to go in front of a group of business representatives and be able to, very succinctly or in a powerful way, say how the data is communicating various movements and trends.
However, while a data analyst would typically only work with structured data that is already collected, a data scientist would also work with unstructured data – be it natural language, text, or images. To work with unstructured data, you have to have an arsenal of tools or skills – such as proficiency in high-level languages like Python – in order to do analysis.
Overall, both roles are very similar. You’re a data practitioner who deals with data on a daily basis. The difference comes in with being mainly focused on either descriptive or prescriptive ways of using that data.
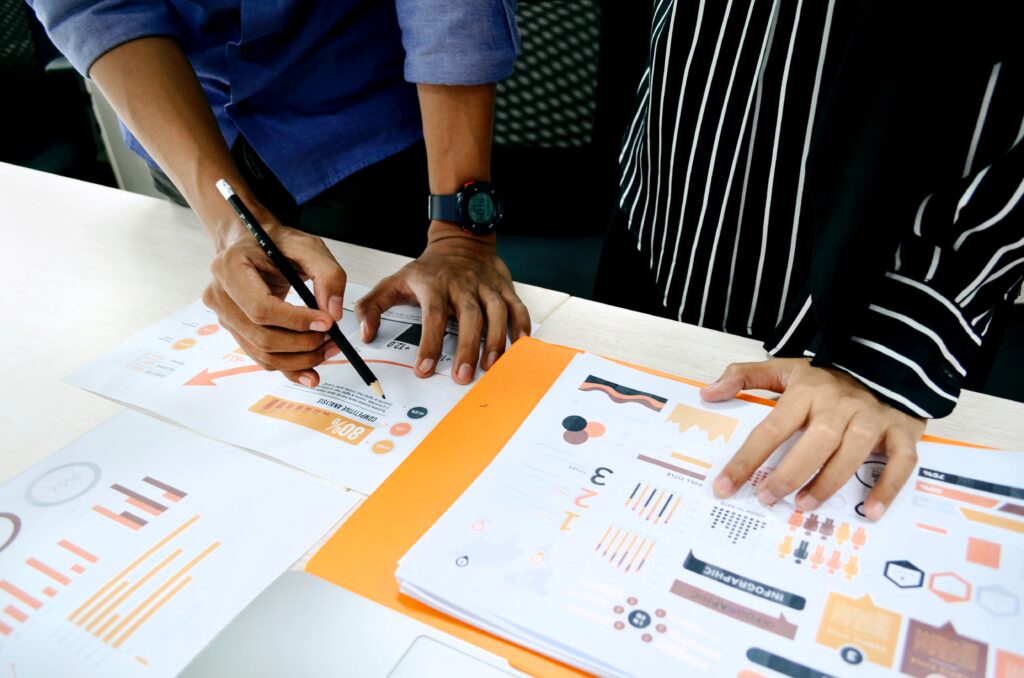
Q: Which field provides more career opportunities?
A: Both have so many career options.
Typically, we see a role in data analytics having a lot of context within a business. As a data analyst, you’ll see yourself being drawn more and more into the working of a business and so you naturally can go along that path.
As a data scientist, the roles also go into business, but you might find yourself specializing as a machine learning engineer, for example, or a data engineer. These are closely-related fields that are exploding at the moment and going forward.
It really comes down to your personal preference. Is it the interaction between a business and its data, from a descriptive angle, that holds your interest, or is it the actual science of the data that intrigues you. Both fields hold gainful futures, so you can’t make a wrong decision.
Q: What does a day in the life of a data scientist or data analyst look like?
A: This greatly depends on the domain you’re working in, as well as the maturity of the business you’re working in.
If you’re in a slightly less mature organization that has realized the value of its data, but only has a small group of data scientists, you might find yourself working with a lot of the raw data at your disposal. You may spend a lot of your time cleaning or engineering the dta, or working on the underlying transformations that are required to make sense of it.
You may also be responsible for creating the data pipelines that move data into a space where it can be analyzed and modeled. This would see you taking on the role of both data engineer and data scientist.
As a data scientist in a more mature company – like ExploreAI – you’ll often find yourself working alongside other data practitioners. You’ll likely be part of a team of data engineers who have streamlined the data into a format that is easier to understand. A data scientist doesn’t just stick their heads down to code and deliver a model to clients. They work together with domain experts who advise on where to focus their attention in a process called incremental modeling. This iterative process involves analyzing data and providing those insights to clients. Ultimately, the client will decide whether the data and insights speak to their end business goal.
Within the product space, teams of data scientists start with general business questions that their clients might have in order to understand what is needed to address those needs. This involves having several meetings with the client and domain/subject matter experts to understand their needs fully. Following this, you then can dive into getting to know the data itself.
It’s very exhilarating to see how different departments interact with the data and iterate the insights according to the client’s business goals.
Q: How much do data scientists and data analysts make per year?
A: This would vary greatly depending on your level of seniority and experience. In general, this is a high-paying profession straight from the jump.
A junior data scientist typically earns around $60,000 USD/year. As you grow in seniority, that can go up quite a bit to over $100,000 USD/year. These salaries are overall very competitive compared to other fields.
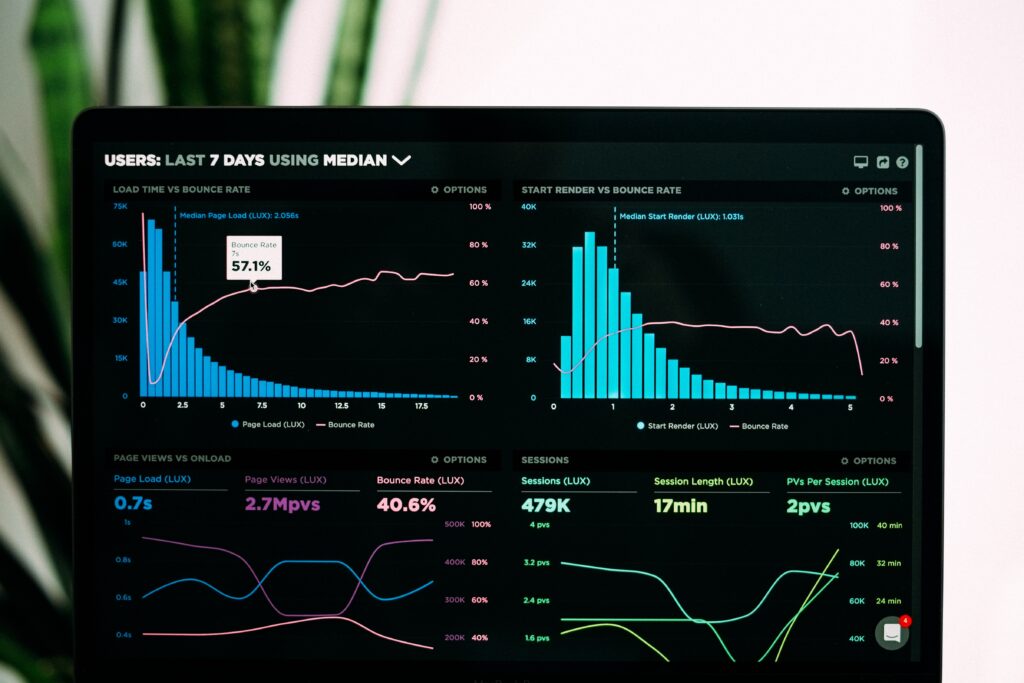
ALX Global, in partnership with ExploreAI, offers cutting-edge courses in Data Analytics and Data Science. Apply to join one of these world-class programs and discover your next big opportunity with a career in data.
Part 2 of this Q&A explores these programs in more detail, with a focus on the lucrative careers they will prepare you for.